Elevate your team's link sharing
Effortlessly share important links with your team, from any device. Get lightning-fast access and streamline your link sharing. Try us now!
Aliases
Set up convenient shortcuts and leverage commands like
/go, any link will be
at your fingertips.
Hit alt + a in our browser extension or simply type
go <alias>
in your browser's address bar.
Replace recurring questions, like “What's the standup link?” with
/go standup.
Powerful shortcuts help you /go faster
- /go standup
- /go docs
- /go jira/core-42
- /go logs
- /go prs/1337
Browser extension
Our browser extension codenamed Omni, is a combination
of a tab switcher and a history search engine with powerful bookmarking
support.
With Omni, team members have instant access to the
team's bookmarks.
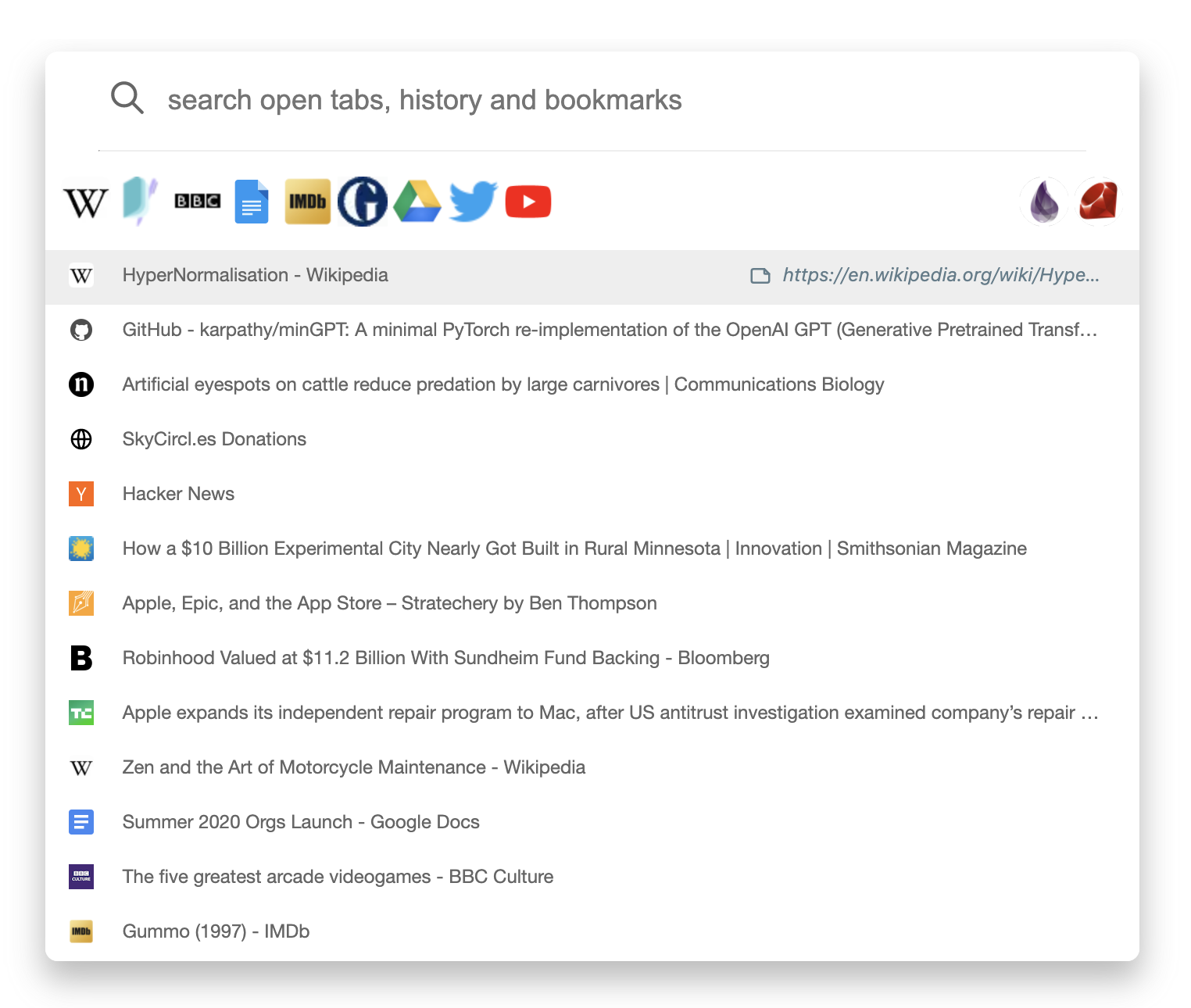
Slack integration
Install our Slack app and members of your workspace can add bookmarks, search and create shorthand aliases for links. One may also invoke an action to scan a message for links and add them as bookmarks.
Install Slack AppCreating aliases
To create an alias use:
/tefter alias <alias> <url>
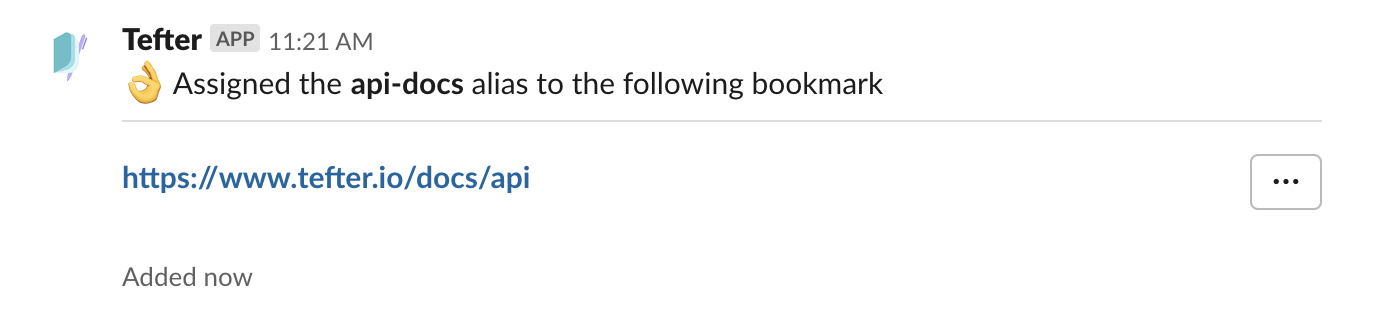
Trusted by
and 60+ more teams
Plans
Standard Team plan
- Unlimited users
- Unlimited bookmarks
- Unlimited lists
- Unlimited aliases
- Bulk bookmarks import
- Search by page content (full-text search)